Ready for the future with Big Data and Artificial Intelligence
For companies and organizations today, everything revolves around data – especially how they process that data. New buzzwords and software […]
For companies and organizations today, everything revolves around data – especially how they process that data. New buzzwords and software technologies emerge in this field every year. The trick is to find the right balance between impulsively embracing each new trend and waiting just the right amount of time before springing into action. If you jump aboard too early, you might be taking too much of a risk – and you’ll most likely be reacting to a hype. Those who wait too long risk missing the boat and are overtaken by the competition. Blindly responding to every new trend and technology is, therefore, not the right way to go.
It’s much more important to develop the right mindset: the knowledge that every single company and organization, both now and even more so in the future, is also a digital company.
Only with that vision will you succeed in bringing people, data and processes together. Because it’s not about software technology per se, but rather the doors that this technology can open. If you have the right data mindset, business processes will run more efficiently, you will gain insights into market developments, strengthen your HR policy and better understand consumer behavior. Ultimately, this increases a company’s customer satisfaction level and competitiveness. With the right digital vision, you will also be able to evaluate trends, experiment quickly and create added value for your organization, employees, customers and partners in all kinds of ways. And that is crucial, because the world will continue to change dramatically in the coming years, and more and more data will be generated.
In this blog, we zoom in on big data:
- What opportunities can big data open up for your organization?
- How can data be optimally processed and analyzed – and even become trends and predictions?
- What’s the best way to approach this? And what mindset and expertise are required?
The answers to all these and many more questions will help your organization navigate its way through a technological shift, tailored to your corporate culture. A switch to more data-driven decisions, processes and entrepreneurship. In short: a shift towards an optimal digital transformation.
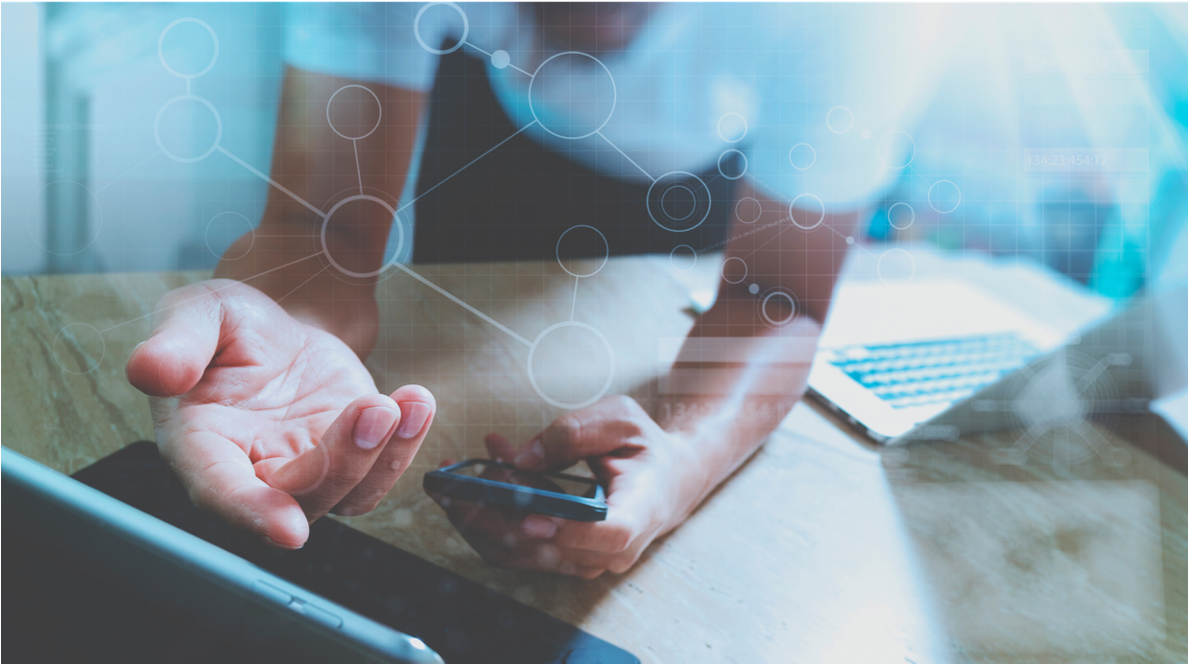
What can companies learn from big data?
Online clothing and shoe retailer Zalando, for example, knows that its web shop is visited 2.5 billion times a year. That 2.76 per cent of those visits lead to purchases. That an average of 64.5 euros is spent on each purchase. And that a customer makes about 3.9 purchases per year. With this strategic data, Zalando can find out who their “ideal” customer is, which products they should offer to that customer, and at which point in time they can do that. This is just one of the many business examples where it is beyond doubt that big data will become increasingly important for companies in the coming years.
This explosion of generated and stored data has not simply happened by chance. In recent years, memory storage devices have become increasingly larger, cheaper and, thanks to the cloud, more accessible and easier. The challenge for companies now is to put all this data to use in an intelligent way; to transform data from a passively stored by-product into an active policy instrument. Some people call data the new gold. Nathan Bijnens and Wesley Backelant agree. As Cloud Solution Architects at Microsoft, they are both experts in the field of smart data processing. ‘These days, every company has a huge amount of data sources’, says Bijnens, who helps the EU and the public sector, among others, to do more with their data. ‘So, it makes absolute sense and is a good idea to look for ways to do something meaningful with this data. The data comes from everywhere; from classic sources such as ERP and CRM systems, databases and Excel files, but also from newer applications such as Internet or Things (IoT) sensors, control systems within production units, click and weblog data etc.’
Dream team: big data and AI
Each company has its own data sources, but simply collecting and keeping track of all that data does not provide any direct added value. This only happens when you process the data in a smart way: individually per data source, or in a group. There are no ready-made solutions for this. Something like this must be customized to suit the needs of each company. What is the same for all companies is that data is growing faster worldwide than people can be trained and called upon to analyses it. In addition, there are many data sources that are only useful when they are processed in real time: data from social media, for example. Therefore, algorithms are indispensable. They are an invaluable part of artificial intelligence (AI). An algorithm is a series of instructions that lead you from a starting point to an end point. Without those instructions, machine learning (techniques with which computers can learn, analyses and even make predictions themselves), for example, would not exist.
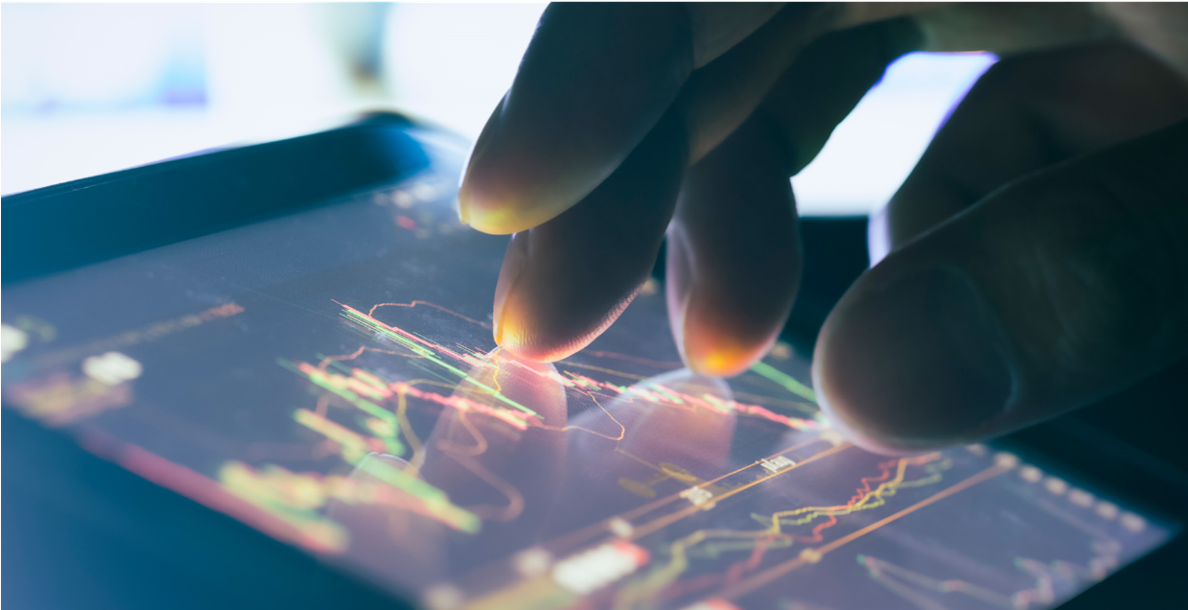
‘Lately we have seen a great deal of innovations in the field of smart algorithms’, says Backelant, who helps industrial and automotive customers with their data processing. ‘These algorithms are becoming increasingly accessible and are also increasingly able to combine and/or span different data sources and computer applications. Also important is the fact that AI has recently made it possible to process certain data sources that until a few years ago were unusable for distilling useful data. This includes photos, video images and speech, which can be analyzed automatically. Today, for example, English speech can even be recorded and understood by machines with a margin of error that is as small as if two people were talking to each other. This so-called human parity, in which computers understand spoken languages just like people, will only increase in the coming years. As I said, this is already happening flawlessly in English. But in the future, this software will not only be able to correctly register and understand more languages, but also accents and pronunciations.’
Know your customer / Know your company
The smart handling and analysis of different data sources provides added value for every company, regardless of the sector in which it operates. First and foremost, companies that really focus on their data get a much clearer and better insight into their customers, according to Bijnens. ‘Companies that better understand their customers can also respond more efficiently to their needs – and in a more personal way. Those who achieve that insight into their customers thanks to a targeted data analysis have an extremely powerful policy instrument at their fingertips. Data insight not only provides knowledge, but also a competitive advantage. It is no coincidence that most companies that challenge or even disrupt the market today are data driven. Just look at Netflix and Facebook. Companies that understand their customers through data can make the right decisions, faster.’
But there’s more. Smart data analyses can also be used to improve and optimize business and production processes. Take AB InBev’s SmartBarley platform for example, Wesley Backelant explains. ‘Barley is an essential ingredient for beer production. The brewery’s digital solution brings together the knowledge of barley growers to create value for their harvests and the supply chain. By having access to crop data collected from all over the world, farmers can learn from each other’s experiences, exchange good practices and learn about new techniques. This way, they acquire new insights and learn from each other. This ultimately results in barley of a higher and more consistent quality. This reduces costs: the harvests are better. But it is also better for the environment because fewer pesticides need to be used. And of course, better barley is also an added value when brewing beer.’
It’s clear that the analysis of big data is so much more than just a technological tale. Analyzing data purely for the sake of it is of very little use. The key is to set goals. As a company, you do this by looking for ways in which data can improve the organization, says Ken Geeraerts. He is BI Team Lead at Kohera, which assists companies in the smart conversion of data into insights. ‘You first have to make sure that the data is stored in the best possible way and then made available centrally. Then you look for ways to convert that data into a policy instrument; that helps you make targeted and strategic decisions.’
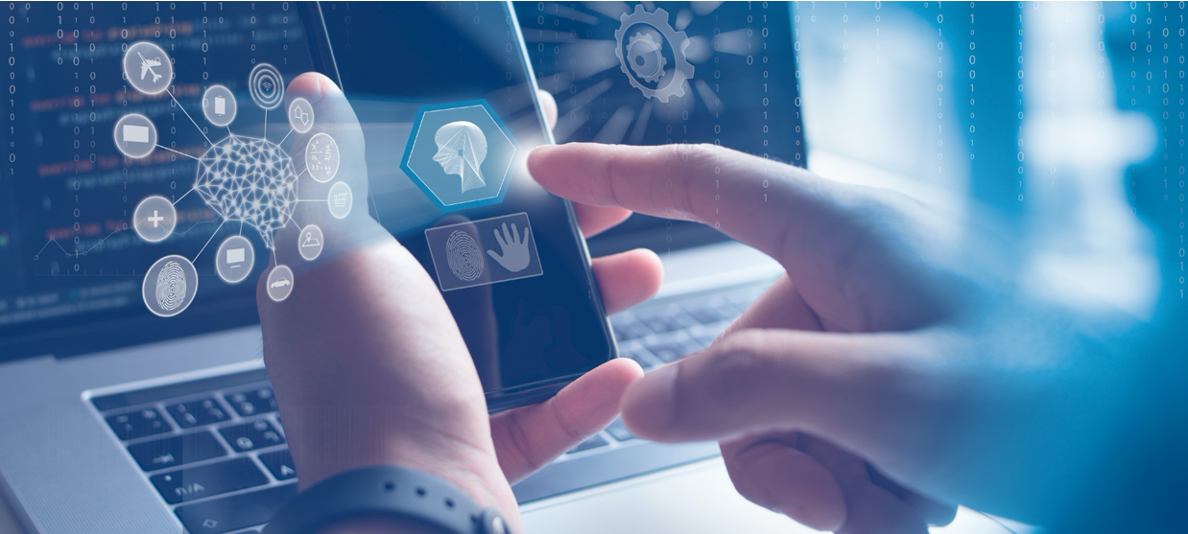
In short: stored data first needs to be activated. This then results in certain interpretations and insights, and from these insights data-driven decisions can then be made. Every company benefits in one way or another from this transformation process. For example, Kohera created a cloud solution for the Kinepolis Group. The cinema giant uses this solution to find out why customers go to the cinema. From there, the service can be even better tailored to the customer, and new products can be launched. In other words: the solution ensures that customers are better understood and served.
Proof of concept: technology is not the end goal
‘When implementing methods to transform data into new information, it’s important to start small’, says Nathan Bijnens. ‘The most important thing for a company is that you get started! Of course, you don’t have to start with a huge project right away: start small. Work on a proof of concept that also provides added value in the short term. Don’t think too theoretically about goals you want to achieve in five years’ time, for which you first need two years of preparation. If you start today, you will start learning lessons from the whole process and build up experience. The feedback that results from such an initial experiment is also invaluable. If you analyse data to be able to make better decisions, or to predict things, it is important that you can measure the impact of that process.’
In this respect, the most successful data companies now are setting a good example: it is no coincidence that each and every one of them has built well-thought-out feedback loops into their data processing. ‘A problem that often recurs in practice at a number of companies is that they do not or hardly want to set aside a budget for data experiments’, Wesley Backelant observes. ‘For this purpose, as well, small start-up projects are an ideal solution: they are the first step on the road to developing the right data mindset for a business. And with their direct results they can also pave the way for larger projects at a later stage: at departmental or company level. It is equally as important to accept that such an experiment can also fail or not produce the desired results. But that, too, is an excellent learning process. And when done on a small scale, it’s not a disaster either: everything can be adjusted fairly quickly and easily. That used to be different. Until a few years ago, AI projects were inherently gigantic. For example, I know a company that has spent years collecting data using all kinds of sensors. A large AI project recently failed, resulting in a lot of time and money wasted. If that company had set up a small experiment at an early stage, they would probably not have had that problem: certainly not on that scale.’
It always makes sense to start small, in both the short and long term. Even within a period of just two weeks, you can achieve a lot with such an experiment, says Nathan Bijnens from experience. ‘You quickly get a pretty good picture of whether you’re on the right track, and whether the approach can be extended to achieve the desired results. This means that just about any medium-sized or large company can start such a big data and AI process without virtually any risks. I think it’s actually riskier not to do such experiments, or to postpone them. How should you get started? Start by identifying the type of data that is generated within the company, and then determine what you want to get out of it. Then set up a pilot project to see how the desired results can be achieved, or how they definitely cannot be achieved. This approach really works. We see that every day with our customers.’
Someone who shares the same opinion is Danny Otten, sales manager at Nimbuz. ‘Building a pyramid that lasts for centuries is a very slow process and it costs a lot of time and money. Go for good enough: a good tent can be put up in no time and lasts long enough. That way you also keep the budgets under control.’
Kohera often kicks off such a process with a workshop, Ken Geeraerts explains. ‘We spend two days identifying the business needs and challenges and how they can be solved. We then select the most urgent topics so that we can come up with a proof of concept. We can then build on that later.’ Danny Otten of Nimbuz also often uses a similar approach. ‘We listen to our clients, and then inspire them. We do this by showing them examples of data solutions that we have built or designed for other clients. From there, we create a project that might also benefit them, and we formulate an initial proof of concept: within a budget-friendly, realistic timeframe.’ The chances of success of an initial data experiment depend, of course, on the complexity of the issue in question. For example, a test with a chatbot system, for which several concrete solutions already exist, will rarely end in failure these days. Today, there are numerous AI models with a proven track record of strength and efficiency, and that were developed by both commercial international companies and as open-source solutions available free of charge. These models can be processed in a very accessible way and embedded in the data flows of companies, says Wesley Backelant. ‘Suppose a company wants to analyse social media channels. In doing so, it wants to go further than simply counting the number of likes and reactions. It also wants to map the content of those reactions or images automatically, while of course respecting privacy regulations. Today there are tested models that can be used straight away. And those models are also becoming increasingly accessible. Moreover, they can be personalised and scaled, tailored to the processes for which companies want to use them. This also saves time and costs.’
The crystal ball: AI as a predictor
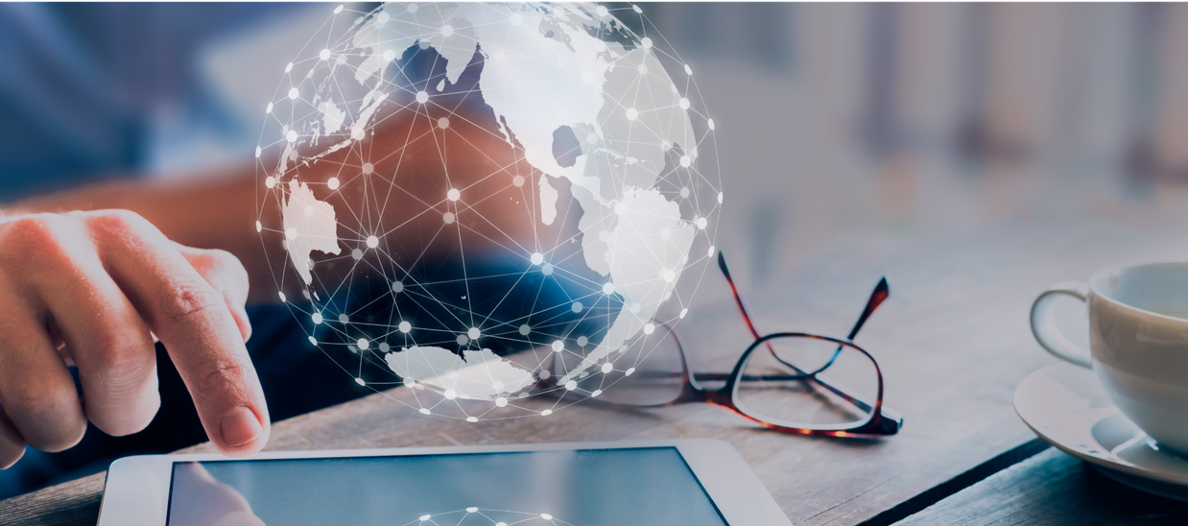
Machine learning can also be used to predict certain trends, evolutions, needs or actions. That, too, will only increase in the coming years. Nathan Bijnens: ‘Predictive maintenance is a good example of this. They are predictive maintenance techniques, usually via IoT sensors, which help determine the condition of equipment. This makes it possible to estimate the best moment to carry out maintenance. This saves costs compared to routine or time-based preventive maintenance, because tasks are only carried out when they are justified. Or advice can be issued automatically, in the field of production quality control, for example. There are also possibilities for marketing companies to predict the number of clicks on a link. Energy companies can anticipate electricity consumption patterns. Fintech companies use these models to proactively trade on expected stock prices. Today, for example, many investment transactions are already fully automated: to save both time and costs. Farmers can use satellite images and weather forecasts to optimise their harvests. NGOs can predict the time and location of outbreaks of disease and epidemics – and thus use these models to save lives, for a better world. The same applies within healthcare, where, for example, certain cancers or other conditions can be predicted via smart data processing. Or AI that uses radiological images to detect conditions at an early stage, which are not yet visible to the human eye. In short: the possibilities for using AI predictions are truly endless.’ Danny Otten of Nimbuz mentions even more examples where data predictions can help companies move forward. ‘We currently have a project in the preparatory phase for a drinks producer. They want to see a correlation between changes in the weather and which drinks are sold the most in which weather type. They can use that information to adapt their production: in warm weather, consumers drink different things than when it is cold, for example. Based on weather forecasts, the producer ultimately wants to fine-tune its purchasing of raw materials and production processes. This will help them optimise their production capacity. Even if they achieve only a 2% improvement here, this will still be an immense return. Especially when the project is rolled out internationally later on. Simply put: the possibilities for using analyses and predictions based on data are almost limitless.’
Without the cloud, there’s no AI
The cloud is a big enabler of AI. A company that wants to start a short-term experiment does not have the time to first develop an entire big data infrastructure for it. Thanks to the cloud, you can get started right away, says Wesley Backelant. ‘This means existing solutions – both commercial and open source versions – can be used straight away to tackle a specific data challenge. The cloud makes AI more accessible and cheaper than ever. In fact: without the cloud, AI would probably not exist today.’
“The cloud makes AI more accessible and cheaper than ever.”
Ken Geeraerts of Kohera also believes that the cloud plays an indispensable role. ‘It makes the process more affordable. Developing and implementing a data solution within a company’s physical IT structure often costs many times more than making it available in the cloud. Scalability in the cloud is also easier, more flexible and cheaper. It is also easier for a company to “quickly” place a gigantic volume of data in the cloud and then run the AI model, which is then properly trained, and from which the right conclusions can be drawn. You can build such a test project in the cloud in just two days and then throw it away completely if you need to, with minimal costs, to try something new and better. That’s impossible to do on-premise. Locally installing an AI solution on an organisation’s computers is simply the old-fashioned way of doing things.’
Digital transformation
A fundamental mistake companies sometimes make is to view and treat big data and AI as an IT project. After all, the role of the IT department within a company has changed dramatically in recent years, and will continue to do so. At best, IT is a partner in this story. A company’s IT department should never be completely excluded when it comes to big data and AI: after all, they have the best knowledge about the company networks, the data stored in them and how everything is secured. ‘The fact is that companies have a huge responsibility when they are working with data’, says Wesley Backelant. ‘Security is extremely important in that regard. A data loss or incident not only causes technical difficulties, but often also reputational damage. A big data project only works when it can also be executed securely.’
“Companies that are not actively working with data in a few years’ time will sooner or later become irrelevant, there’s no doubt about that.”
Some companies have data, but don’t know what to do with it. Other companies don’t even realise that they’re sat on a mountain of useful data and information, explains Danny Otten. ‘When they analyse their clients’ data in a targeted way, they discover what information can be extracted from that data. For example, the number of hours worked on a fixed-price project. And we often see this in production environments as well, where a lot of data is generated by machines and sensors. These data are often isolated from each other. By pooling them and combining them, you generate a lot of new insights. You can then use these, for example, for more optimal planning of production processes, the purchase of raw materials and deliveries, and the deployment of personnel. Usable data is everywhere.’
In any case, your big data and AI will be more effectively managed in a business transformation process. You can set up a data analysis department or an innovation lab for that purpose. Or implement it even more extensively, for example, by introducing a data scientist to each business unit. Nathan Bijnens: ’This makes organisations very agile when it comes to responding to changes: they can anticipate them or even innovate in certain areas. Data not only strengthens and accelerates companies, but also makes them more resilient. Companies that are not actively working with data in a few years’ time will sooner or later become irrelevant, there’s no doubt about that. Data analyses are becoming less and less an option, and more of a necessity. Those who don’t do it, will be beaten by players who have done their homework, and therefore have gained an advantage.’
Start today, be ready for tomorrow
The future? General AI: The findings and insights gained through big data can also be applied to other domains. A pre-trained model that can be enriched with your own company data. A company’s advantage will be less and less based on the technology used, and more on the data they process with it. The feedback loop they get from it, and how they strategically use the results to make decisions. Ken Geeraerts of Kohera believes that big data in combination with blockchain and the Internet of Things (IoT) will be able to measure or predict a kind of butterfly effect in a few years’ time. ‘For example, it will be possible to predict that when a customer buys a bottle of milk in shop X, there is a good chance that they will book a trip to Senegal next year. More and more connections will be made that seem impossible today. The options available to companies to improve their activities, strategies and results are truly endless.’
In the coming years, extremely powerful quantum computers will further strengthen and accelerate AI’s role within big data. This will enable more and more real-time analyses and predictions to be made, says Ken Geeraerts. ‘Thanks to quantum computers, data streaming will be capable of streaming analytics. Those computers already exist. And they will probably also become more accessible and affordable in the future. ‘Danny Otten agrees: ‘These powerful quantum computers pave the way for real-time decision-making. In the past, computers used to make calculations; today and certainly tomorrow, they will help to make decisions. ‘AI will certainly be much more accessible, believes Nathan Bijnens: ‘That trend is already picking up steam. Look at chatbots: they already represent a supple collaboration between humans and algorithms. I can also envisage AI being used more in smarter assistance and RPA: Robotic Process Automation, in other words the automation of repetitive actions via software. But also being able to look up and retrieve information is crucial in a data processing strategy. Many of the organisations I work with could no longer see the wood for the trees because of the large number of archives they have created. These organisations can be helped with a much smarter search on their own documents. Gaining access to the right information at the right time accelerates business processes. In this respect, I can see many exciting innovations in terms of text analyses, speech, search terms and chatbots in the coming years.’
“Gaining access to the right information at the right time accelerates business processes.”
When is a digital transformation considered a success?
When the user sees that there is a Return On Investment (ROI), concludes Ken Geeraerts. ‘They will notice this automatically, when they can act and make decisions faster, better and in a more targeted way. And they won’t need to rely as much on their gut feeling, but more on objective and optimally substantiated analytical data, to which they have access via a clear, comprehensible dashboard.’ A digital transformation should not be a project; it should form part of the company’s overall strategy. Danny Otten: ‘Companies should develop a compatible vision for this. You can’t draw up a plan for the next three years, because this is a dynamic process. But as a company, what you can do is make sure that everything you do originates from out-of-the-box thinking. That is how you develop a general vision. Otherwise they will remain separate projects and will never form a coherent whole. As a company, you will have frittered away a lot of potential.’
In addition, actually initiating a digital transformation – getting started in the first place – is more important than the end goal, thinks Nathan Bijnens. ‘It is vital that you are ready to make the transformation. But a digital transformation is, by its very nature, never complete. As a company you have to keep continuously evolving, just like the markets in which you operate. The end goal of a digital transformation is to create an organisation that is constantly changing. One that is ready for change, and can respond to that change in the best way possible. It can’t do that with technology alone. It also needs to have the right mindset, so that it understands that next year’s business world may be completely different from this year’s. It should be able to anticipate that development by analysing and even predicting data. Companies can only ever achieve all those things by making a start. Preferably sooner rather than later.